Identifying keystone species in microbial communities using deep learning. Nature Ecology & Evolution (Published: November 16, 2023). The paper can be downloaded here.
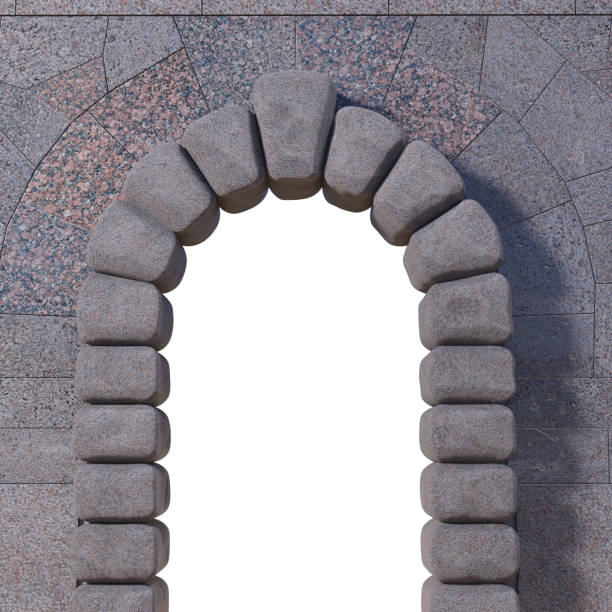
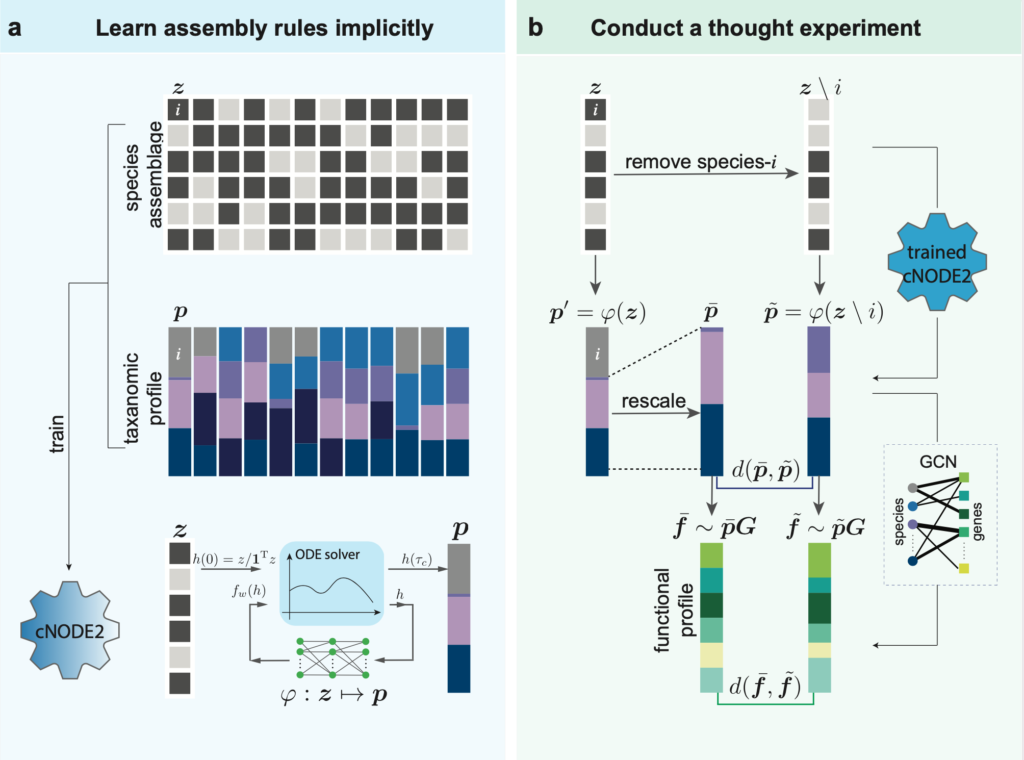
Previous studies suggested that microbial communities can harbor keystone species whose removal can cause a dramatic shift in microbiome structure and functioning. Yet, an efficient method to systematically identify keystone species in microbial communities is still lacking. Here, we propose a Data-driven Keystone species Identification (DKI) framework based on deep learning to resolve this challenge. Our key idea is to implicitly learn the assembly rules of microbial communities from a particular habitat by training a deep-learning model using microbiome samples collected from this habitat. The well-trained deep-learning model enables us to quantify the community-specific keystoneness of each species in any microbiome sample from this habitat by conducting a thought experiment on species removal. We systematically validated this DKI framework using synthetic data and applied DKI to analyze real data. We found that those taxa with high median keystoneness across different communities display strong community specificity. The presented DKI framework demonstrates the power of machine learning in tackling a fundamental problem in community ecology, paving the way for the data-driven management of complex microbial communities.
Media report:
https://bwhclinicalandresearchnews.org/2023/11/13/whats-new-in-research-november-2023/#liu
https://phys.org/news/2023-11-framework-keystone-microbial-species.html